Notice
Recent Posts
Recent Comments
Link
일 | 월 | 화 | 수 | 목 | 금 | 토 |
---|---|---|---|---|---|---|
1 | 2 | 3 | 4 | 5 | ||
6 | 7 | 8 | 9 | 10 | 11 | 12 |
13 | 14 | 15 | 16 | 17 | 18 | 19 |
20 | 21 | 22 | 23 | 24 | 25 | 26 |
27 | 28 | 29 | 30 |
Tags
- pymysql
- 파이썬 내장 그래프
- 범주형 변수 처리
- paper review
- 데이터 분석 플랫폼
- 브라이틱스 서포터즈
- 서포터즈 촬영
- Deep Learning for Computer Vision
- Brightics AI
- 분석 툴
- 파이썬 SQL 연동
- Brightics 서포터즈
- 데이터 분석
- Random Forest
- 머신러닝
- 브라이틱스 AI
- 브라이틱스 분석
- Activation Function
- 브라이틱스 스태킹
- 딥러닝
- Brigthics Studio
- 삼성 SDS
- 브라이틱스 프로젝트
- Brightics EDA
- 삼성 SDS 서포터즈
- 검증 평가 지표
- Brightics studio
- 비전공자를 위한 데이터 분석
- Python
- michigan university deep learning for computer vision
Archives
- Today
- Total
목록GBM 이론 (1)
하마가 분석하마
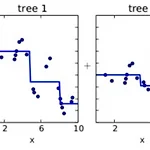
[Gradient Boosting Machine] 1. Gradient boosting = Boosting with gradient decent 2. 첫 번째 단계의 모델 tree1을 통해 Y를 예측하고 Residual을 다시 두 번째 단계 모델 tree2를 통해 예측하고, 여기서 발생한 Residual을 모델 tree3로 예측 3. 점차 residual이 작아짐 4. Gradient boosted model = tree1 + tree2 + tree3 => residual을 계속 모델링하여 나오는 아이디어를 모으는 것 [gradient 의미] - 미분이라 생각 - Loss function에 gradient는 손실함수를 f(x)로 미분한 값 => residual이 결국 gradient를 의미 따라서 처음 ..
알고리즘
2021. 3. 8. 00:35